The Future of Medical Data Annotation: Enhancing Precision in Healthcare
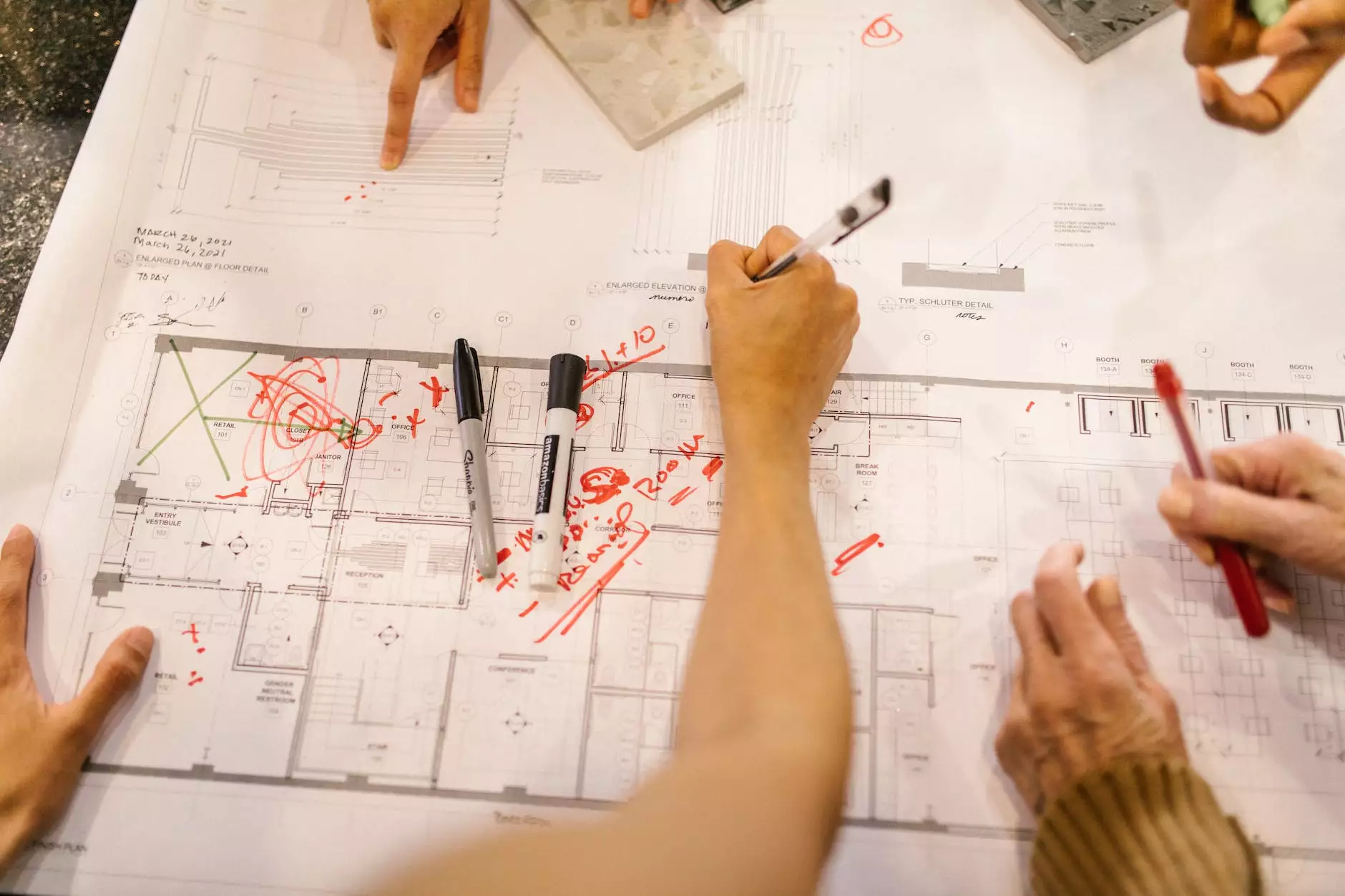
Medical data annotation is increasingly becoming a cornerstone of innovation in the healthcare sector. As technology continues to advance, the accuracy and quality of medical data have become paramount to improving patient outcomes. This article delves into the intricate world of medical data annotation, examining its significance, methodologies, and implications for the future of healthcare technology.
Understanding Medical Data Annotation
At its core, medical data annotation refers to the process of labeling and categorizing medical data for machine learning and artificial intelligence applications. This data encompasses various forms, including images (X-rays, MRIs), texts (clinical notes, medical literature), and structured data (patient records, lab results). The goal is to provide clear and accurate tags that machines can “understand”, facilitating effective learning processes.
The Importance of Medical Data Annotation
With the burgeoning field of artificial intelligence (AI) in healthcare, the accuracy of medical data annotation is critical. Here are some of the key reasons why:
- Enhances Diagnostic Accuracy: Well-annotated data improves the ability of machine learning algorithms to recognize patterns, leading to more accurate diagnoses.
- Facilitates Personalized Medicine: Accurate data annotation aids in tailoring treatments to individual patient needs, enhancing overall treatment efficacy.
- Drives Research and Development: Researchers can leverage annotated datasets to discover new trends and insights, leading to breakthroughs in medical research.
- Reduces Operational Costs: By automating data analysis through accurate annotations, healthcare organizations can significantly cut down on administrative costs.
The Process of Medical Data Annotation
Understanding how medical data annotation is performed is essential for grasping its impact. The process generally involves several systematic steps:
1. Data Collection
The first step involves gathering medical data from various sources including records, imaging systems, and clinical research data. This data serves as the foundation for the annotation process.
2. Data Preprocessing
Before annotation can occur, the data must be preprocessed to ensure it is clean and organized. This may involve tasks such as removing duplicate records, correcting inaccuracies, and formatting the data appropriately.
3. Annotation Methodologies
There are several methodologies employed in medical data annotation:
- Manual Annotation: Trained professionals review and label data manually. This method, while time-consuming, often ensures the highest accuracy.
- Automated Annotation: Algorithms rapidly annotate data based on pre-defined rules. While faster, this method may require manual verification to ensure accuracy.
- Crowdsourcing: This involves utilizing a distributed workforce to label data. Although cost-effective, it can sometimes compromise on quality if not supervised adequately.
4. Quality Assurance
Quality assurance is a critical component of the annotation process. Techniques such as cross-validation, where multiple annotators label the same data, help maintain high-quality annotations.
5. Integration into Machine Learning Models
Once data is annotated and verified, it is integrated into machine learning algorithms to train models capable of making insightful predictions and decisions based on new, unannotated data.
Challenges in Medical Data Annotation
Despite its many benefits, medical data annotation is not without challenges. Some of the notable obstacles include:
1. Complexity of Medical Terminology
Medical data is often filled with complex terminologies and abbreviations that require extensive knowledge to annotate accurately. This necessitates a workforce with specialized training.
2. Variability in Data Sources
Data collected from various institutions may differ in format, quality, and structure, making standardization difficult. Overcoming this variability is essential for consistent annotations.
3. Ethical Considerations
Using patient data for annotations raises ethical issues, particularly regarding patient privacy and consent. It is critical to ensure compliance with regulations such as HIPAA in the United States.
Future Trends in Medical Data Annotation
The future of medical data annotation looks promising with several emerging trends likely to shape the industry. Here are some anticipated developments:
1. AI-Driven Annotation Tools
With advancements in AI technology, we can expect more sophisticated annotation tools that employ deep learning techniques, reducing the need for extensive manual intervention.
2. Increased Use of NLP
Natural Language Processing (NLP) is becoming an integral part of medical data annotation, especially for textual data. NLP tools can help extract vital information from unstructured sources like doctor’s notes.
3. Focus on Real-Time Annotation
Future systems may incorporate real-time annotation, allowing healthcare professionals to annotate and analyze data on the fly, greatly enhancing decision-making processes.
4. Collaborations and Shared Datasets
As healthcare organizations recognize the value of shared knowledge, collaborations for creating unified annotated datasets are likely to increase, fostering innovation across the industry.
Conclusion
In summary, medical data annotation is a critical element in the evolution of healthcare technology. As we move towards more personalized and efficient medical solutions, the accuracy and quality of annotated data will be pivotal. Organizations like Keymakr.com are at the forefront of this transformation, providing essential tools and services in software development tailored for healthcare applications. Embracing the practices of effective annotation not only drives advancements in technology but also ultimately enhances patient care and health outcomes worldwide.